Is it unfair to say that, when it comes to learning measurement, the collective thinking of the learning community can be summed up in just two metrics? The first extremely pragmatic measurement is learner completion, and the second, mostly an aspiration, is the return on investment.
If you’re sighing, it’s because you’re well aware of the compromise we made with learning management systems to enable the interoperability and distribution of digital learning. Although there was backlash several years ago, the movement to fire your LMS didn’t herald a new era. Mostly, the practical advantages of distribution and tracking outweighed other considerations. Of course, there has been change, and it’s definitely unfair to place all learning platforms into the same category. The promise of next-generation platforms, particularly “integrated platforms,” do more than track completion. So, while it’s tempting to give you 50 ways to leave your LMS (thank you, Paul Simon), leaving really isn’t the point. Maybe the point is more of a vanishing point — it’s what lies ahead in the distance.
What isn’t new is adaptive learning. The research behind adaptive learning pre-dates artificial intelligence that can enable it today. AI isn’t needed to create adaptivity, but it is needed to achieve scale. True adaptivity, with or without technology, factors in a complex set of variables.
The research is diverse — and spans decades — and is dynamic. It goes beyond Bloom’s taxonomy to include his research into the efficacy of one-on-one tutoring over classroom instruction. It is informed by the psychological states in learning progression, the zone of proximal development, the value of interleaving, the criticality of self-efficacy and the inverse logic of the Dunning-Kruger effect. It embraces the science of expertise and the science of individuality.
With adaptive learning, we can apply, test out and optimize our approaches using a much broader toolkit. The most advanced platforms evaluate and measure outcomes going far beyond the practicality of completion or the existential anxiety of ROI.
The data from standard e-learning consists of completion and maybe a score. Mature adaptive learning provides detailed, micro-level analytics. That value is sometimes lost in the terminology that we use to describe adaptive or personalized learning. It doesn’t suggest the real full possibility of a data-driven learning strategy. Here are ten uses for learning data that can shape your learning strategy today.
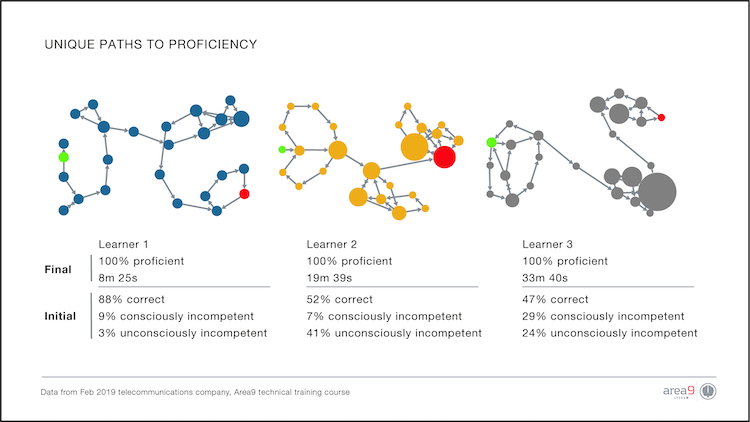
No. 10: Scaffold the learning experience
We tend to monitor learner progress in digital learning as a binary — did they or didn’t they complete the assignment? However, if we assess progress at a more granular level (as micro as every one to two minutes), we can note which learners haven’t started, which have stalled, where they have stalled, how much time they are taking (when and on what objectives) and how well they are performing as they progress. For instructors attentive to the data, it allows for personalized remediation for that learner. If such data is available to the algorithm of an adaptive learning platform, it can be used in real-time to accelerate individual learning.
No. 9: Assess and focus on needs
To understand and prioritize learning needs, we interview subject-matter experts, talk to stakeholders and consider the organizational dynamics. We invest in what’s important to leadership, usually based upon a loose anecdotal connection to individual or group needs. What if — before building out that media-rich learning extravaganza — we quickly curate an agile, minimally viable product to gather data on what learners already know, their awareness of their own knowledge and the time and effort it takes to reach proficiency?
The data might show, for example, that a sales team understands the basic ethics policy, but is largely unaware of how manipulation of a deal is ethically problematic. Rather than treating all content consistently, or comprehensively, a data-driven strategy targets actual rather than perceived needs. In this case, the subsequent, data-informed design of the program might augment the content on sales manipulation with expanded objectives, peer-review activities, scenarios and more developed learning resources.
No. 8: Engage learners
It’s possible that edutainment and learner marketing increase attention without increasing effectiveness. We would not usually equate engagement with effort, but we know that practice, particularly serial practice, leads to expertise. Effort can be unappealing. We’ve been conditioned to surf e-learning like we surf the web, without commitment. A data-driven strategy based on formative practice scales back on content an individual already knows (and is aware of) while expanding on objectives they either don’t know (or thought they knew). In this case, measuring competency removes content that bores learners or gives them false confidence while engaging learners in meaningful practice.
No. 7: Increase efficiency
Is it faster? Comparing typical e-learning to data-driven learning is apples and oranges. The norm does not assess, uses quick checks and may have a final quiz (with an approximate 80 percent pass rate). There is little predictive validity to the quiz and the passing score fast-tracks completion. The seat-time or duration can vary significantly. Comparing seat-time in a typical course to one that foregrounds formative practice begs for several disclaimers. Generally speaking, however, the personalized experience will usually accelerate average training time by approximately 50 percent. How? By minimizing the time spent on what is already understood, more time and resources become available for what may be misunderstood.
Surprisingly, in adaptive learning, there is usually a much greater spread in the amount of time required by different learners (as much as four times the difference in the time needed to gain proficiency). With traditional e-learning, the metric of time-to-completion may only indicate one’s ability to navigate slides or pages. A metric of time-to-proficiency, by contrast, demonstrates the efficiency of individualized instruction.
No. 6: Ensure mastery
A program that measures the path to initial proficiency can also leverage those metrics to inform a reinforcement strategy that remembers the performance of individual learners. Instead of spacing generic content or summarizing key points, a data-driven approach to reinforcement allows for smart curation of precisely those micro-objectives with which learners had previously grappled. Reinforcement can also be queued up based on when the individual completed the previous module and not on a standard, group cadence. On one adaptive learning project, a test group retained 35 percent more knowledge after 10 weeks with personalized reinforcement, compared to the control group.
No. 5: Improve mindset
Learning to learn can be described as self-efficacy, as having a growth mindset or as meta-learning. A practice-based strategy strengthens not only learners’ content-specific knowledge, but their ability to do quality practice in other contexts. In “Four-Dimensional Education,” authors Charles Fadel, Maya Bialik and Bernie Trilling note that not only does developing metacognition help us learn to learn, it helps us to “transfer competencies across disciplines.”. A learner’s successful completion may increase their confidence, but a learner who understands how they are learning, that becomes aware of their misconceptions and that sharpens their focus and attention will develop an expanded capacity for performance on the job.
No. 4: Analyze talent readiness
Another ongoing discussion in our industry is the relevance of the ADDIE model. Just one of the problems of this model is that it leads us to generalize in the analysis phase and to base a learning design on that generalization. We may even create a persona to represent the average learner. Todd Rose lays out the many fallacies inherent in that approach and in that tradition. He calls for a science of the individual that privileges analysis of the individual prior to aggregating data on the group. In the past, we haven’t had good tools for doing such an approach. By understanding individuals first, we avoid the trap of assuming that the generalization applies to all. But the aggregate data will give us a very detailed map of the overall readiness of the organization. In light of the current demand for skilling and reskilling, this aggregate view becomes strategic for the business.
No. 3: Verify learning and mitigate risk
If we’re mitigating risk by displaying content and asking learners to confirm they have read it, then we haven’t really mitigated risk at all. We’ve papered it over. Measuring a learner’s path to proficiency provides granular data on where there may be real misconceptions. That data can be used to correct and reinforce accurate knowledge. An e-learning quiz with a passing score of 80 percent doesn’t provide sufficient assurance. In high-stakes environments with dangerous systems or where mistakes can be costly or have outsized negative consequences, granular data that shows where there may be risks and provides detail on when and how they were remediated can not only save money but can save lives. This is the reason why industries needing verifiable mastery are leading out in terms of data-driven learning. In the life sciences, in the defense industry, in finance and regulated environments, the need for evidence is fueling innovation.
No. 2: Connect to business analytics
Learning and content analytics can be powerful but combining, comparing and correlating that data with that from other business systems and the resulting business intelligence can answer the types of questions with which we’ve struggled in the past. Does customer learning really impact their use of our product? What is the root cause of an organizational challenge? How might growth be limited or expanded based on talent readiness? The question of ROI is too easy. Knowing how to calibrate our solution to address issues of organizational resiliency, readiness or untapped opportunities is much more valuable and is only possible with shared data.
No. 1: Transform your learning organization
Perhaps we’ve already maximized the advantages of small incremental improvements. Those small wins are easier, more practical and more characteristic of our resourceful professional community. In contrast, a data-driven learning strategy demands imagination and fairly radical transformation. This particular change doesn’t easily build on what we’re already doing. It means shifting from a standard experience designed for an average learner to a unique, individual experience. It means displacing the linear, content-first approach with smart practice. Instead of click-for-content and a quiz, we need formative assessment with highly detailed metrics that go beyond right or wrong.
Transformation will require a shift in our group mindset. Data-driven design strains stereotypical binaries of creativity and engineering. It reminds us of the half-life of our accumulated knowledge as professionals. The promise, however, of what might be ahead, reinvigorates the why that motivates us — first as individuals and then as a community.